Adventures in Big Data Analysis
Using Astronomical Methods to Understand Urban Housing Complexities in Baltimore
Tamás Budavári
Assistant Professor of Applied Mathematics & Statistics
The Johns Hopkins University
Video
You can also watch this video on the PSW Science YouTube Channel
Videography by Nerine and Robert Clemenzi, Edited by Nerine Clemenzi Copyright © Philosophical Society of Washington. All rights reserved.
About the Lecture
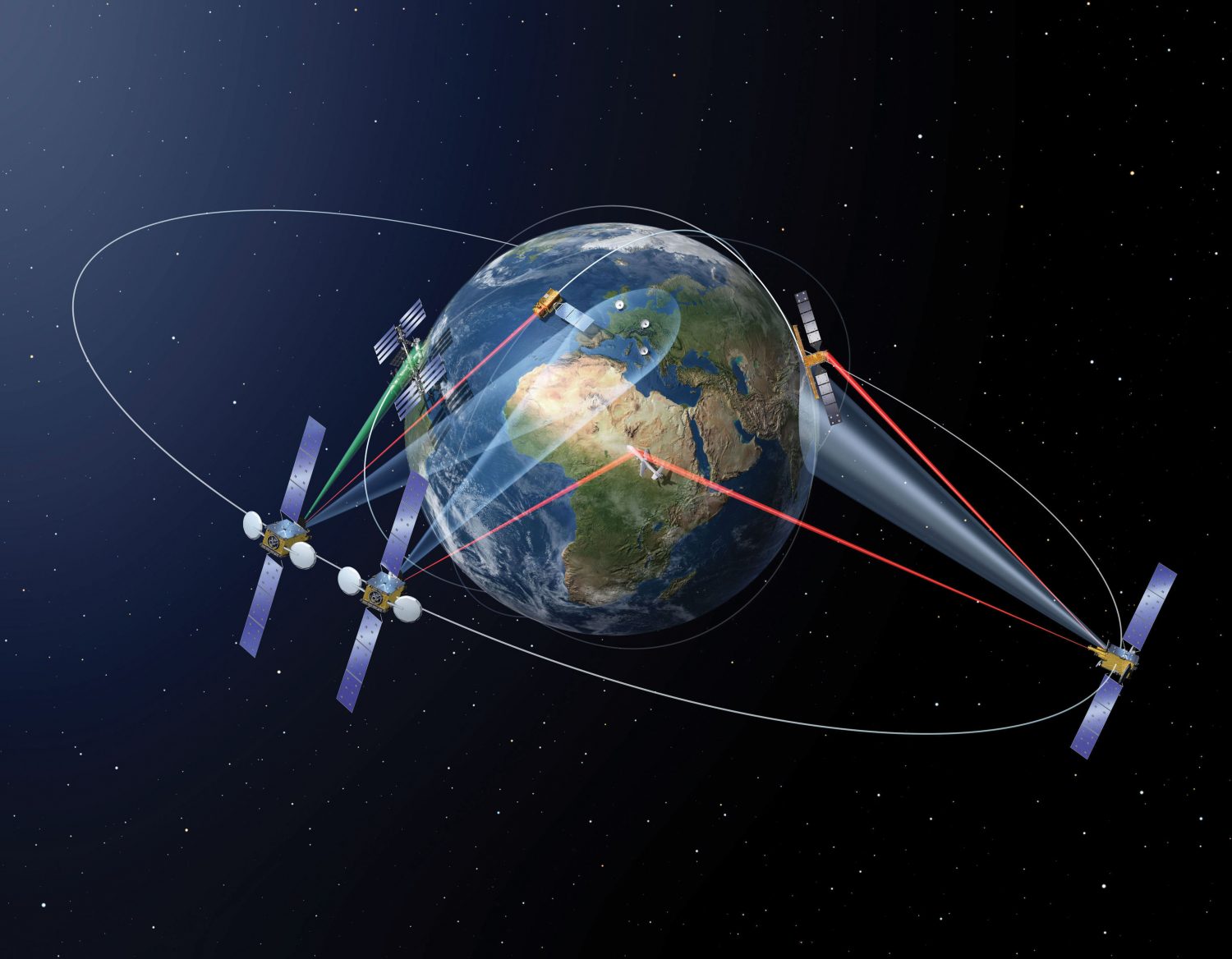
Advances in open data, technology, and geospatial analysis have opened up new possibilities for cross-disciplinary research that blends mathematics and social science. One examples involves applying methods developed for analyzing extremely large astronomical data sets to urban datasets to develop better understanding of housing patterns and building abandonment, with the goal of crafting better policies to prevent urban decay and encourage productive utilization of existing housing stock.
As it happens, remarkably little is known about the dynamics of housing divestment and reinvestment. Fundamental questions have not been answered, such as: When and why are particular properties abandoned? Are there early markers of abandonment that can be identified – before a property begins to deteriorate? If a property is renovated, stabilized, or demolished, how will it effect quality of life and property values in the affected area?
We applied methods originally developed for astronomy to build a unique database that can “ingest” spatial and temporal urban data sets, and effortlessly fuse disparate records on the fly, so that can easily be interrogated collectively for exploratory and hypothesis testing studies. Building on a growing collection of datasets and tools, we are analyzing the spatial distribution of vacant buildings in Baltimore, and we are applying machine learning methods to predict abandonments. We are preparing for a massive combinatorial optimization to find city-wide global strategies that can be translated into practical design scenarios to prevent abandonment and promote productive utilization of urban building stocks. Our project is just one example of how big data tools developed in one discipline often find applications in a diversity of other fields.
About the Speaker

Tamas Budavari is an assistant professor in the Department of Applied Mathematics & Statistics of the Whiting School of Engineering at the Johns Hopkins University. He focuses on the mathematical and computational challenges of big data.
Tamas is the Builder of the Sloan Digital Sky Survey and of its data solution, “SkyServer”. He also created Hubble Space Catalog, a searchable collection of all Hubble Space Telescope observations.
Among other awards Tamas, is the recipient of Statistics and Applied Mathematical Sciences Institute Gordon and Betty Moore Fellowship.
He earned an MS in Physics and a PhD in Astrophysics from Eotvos Lorand University, Budapest, Hungary.
Minutes
President Larry Millstein called the 2,393rd meeting of the Society to order at 8:10 p.m. in the John Wesley Powell Auditorium of the Cosmos Club in Washington, D.C. He announced the order of business, that the evening’s lecture would be livestreamed on the internet, and welcomed new members to the Society. The minutes of the previous meeting and the lecture by Yuval Grossman were approved without correction.
President Millstein then introduced the speaker for the evening, Tamás Budavári, Assistant Professor of Applied Mathematics and Statistics at Johns Hopkins University. His lecture was titled, “Adventures in Big Data Analysis: Using Astronomical Methods to Understand Housing Patterns for a Better Baltimore.”
Baltimore’s population has fallen from a peak 1 million residents in 1950, to approximately 600,000 in this decade, and Budavári believes that abandoned, vacant houses are a principal contributor to urban blight in the city. Conservatively, there are 16,500 such houses in Baltimore today.
To address this problem, Johns Hopkins University turned to Budavári and his background in applying methods for analyzing large astronomical data sets.
Budavári began by creating an interdisciplinary team at Johns Hopkins and partnering with the City of Baltimore to gather the relevant data sets, including geospatial information for all parcels of city land and time history of real properties. The team was tasked with recommending how the city should allocate its budget to most effectively improve quality of life by addressing its vacant house problem.
Budavári’s team first categorized the data by neighborhood, allowing the team to identify neighborhoods that were similar to each other at present and at historical points in time. Budavári said that identifying these relationships allows analysts to control for area-specific public policy implementation.
The team identified correlations between crime, property values, and proximity to vacant houses. These correlations were stronger when the vacant house was collapsed. Their analysis further showed that demolishing these houses correlated to improving nearby property values.
In Baltimore City, row houses are predominant and Budavári’s team discovered that vacant houses at the ends of a row of houses are at particular risk for collapse. The team identified 3,000 such houses, and within a month the city had demolished 400.
Budavári’s team then used big data analysis techniques to identify which non-collapsed vacant houses the city could demolish to provide the greatest overall impact in quality of life for city residents. They also recommended potential property-use conversions for the demolished properties, for example, rehabilitating the property into new housing or an urban park.
The team’s current work is in predictive analytics. One team member, doctoral student Phil Garboden, explained the team’s use of the city’s large historical data sets to identify un-occupancy as the greatest predictor of abandonment spikes in any given neighborhood. As a practical matter, obtaining accurate un-occupancy data is the biggest challenge to honing the team’s predictive model.
Budavári concluded by saying new technologies such as satellite imagery will provide more accurate data to allow the city to more effectively address its vacant housing problem.
President Millstein then invited questions from the audience. After the question and answer period, President Millstein thanked the speaker, made the usual housekeeping announcements, and invited guests to join the Society. At 9:52 p.m., President Millstein adjourned the 2,393rd meeting of the Society to the social hour.
Weather: Mostly Cloudy
Temperature: 29.5°C
Attendance: 99
Respectfully submitted,
James Heelan
Recording Secretary