The First Serious Problem Solved by AI
How Proteins Fold to Become the Machines of Life
John Moult
Professor
Institute for Bioscience and Biotechnology Research
University of Maryland
About the Lecture
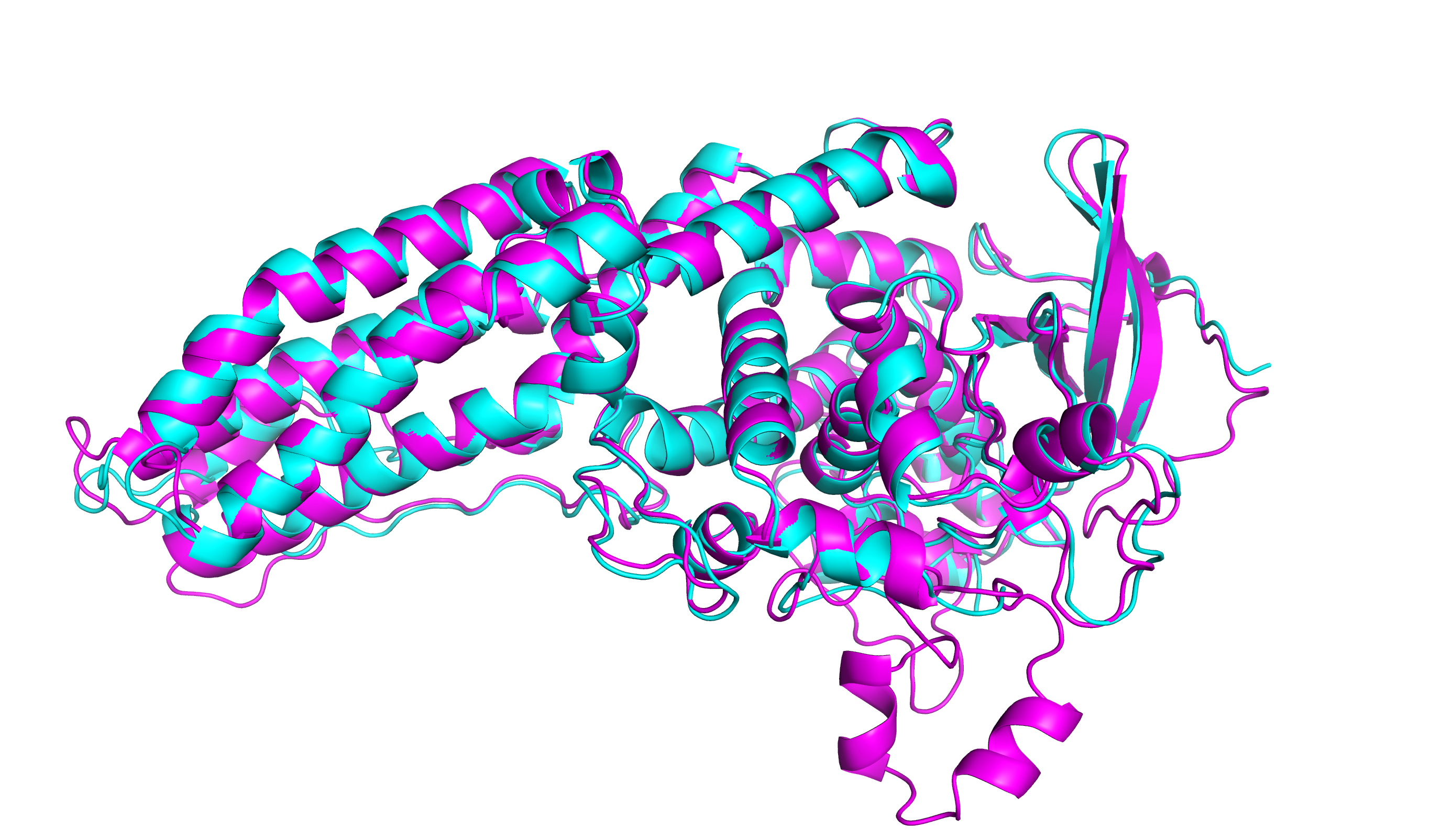
Artificial Intelligence is beginning to have an impact on our lives. So far, the impact is small. This talk will discuss the first serious problem to be solved with this technology – how protein molecules fold into their functional shapes. Genes code for proteins, and each gene’s sequence of DNA bases is translated into a polymer of 20 different sorts of amino acids. The sequence of amino acids is such that most proteins fold into a compact shape, in which each of its 1000s of atoms has an ordered position relative to all the other atoms. The number of possible structures (shapes) these extraordinary objects can adopt is super-astronomical, and the problem of computing structure from amino acid sequence has been one of the unsolved grand challenges of biochemistry for more than half a century.
Progress in the field has been advanced by a series of community experiments, aimed at maximizing rigor, transparency, and communication. Many methods have been developed, tested and discussed. However, while progress had been made and the field advanced, none of the approaches that have been developed has had more than partial success. At the most recent community experiment dramatic results were obtained showing that the program has largely been solved – by a branch of AI called Deep Learning. The calculated structures obtained by the Deep Learning methods are often comparable, and likely sometimes better, than representations to those obtained with state-of-the-art experimental techniques of crystallography and cryo-electron microscopy. The Deep Leaning protein models have already demonstrated an ability to solve previously intransigent experimental problems, and the results suggest the methods will be successfully applied to other areas of structural biology and more generally.
This lecture will describe the intellectual journey to this solution, the nature of the methods used, and some implications for the future of AI and its applications and effects on sciences and other areas of human endeavor.
About the Speaker
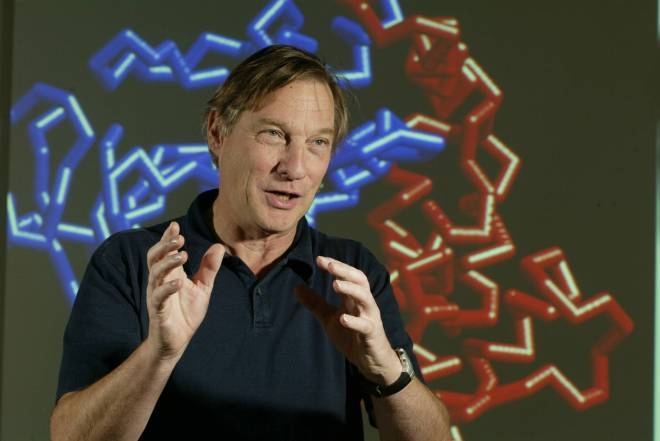
John Moult is a Fellow at the Institute for Bioscience and Biotechnology Research and a Professor in the Department of Cell Biology and Molecular Genetics at Maryland. Previously he was a Founding Fellow of the Center for Advanced Research in Biotechnology at the University of Maryland. John did postdoctoral work at the Weizmann Institute of Science and at the University of Edinburgh, and he was then on the faculty at the University of Alberta, Canada before coming to the University of Maryland.
John is a leader in the development of new approaches to community science, aimed at maximizing rigor, transparency and communication, particularly in computational biology. He founded the Critical Assessment of Structure Prediction (CASP) to advance methods for computing protein structure, and he co-founded the Critical Assessment of Genome Interpretation Assessment (CAGI). He has also been instrumental in establishing several other community science programs, including DREAM (systems biology), CAPRI (protein-protein docking), SBV (quality assurance in industry research pipelines), and CACHE (drug design and discovery).
John’s early research was in the use of X-ray crystallography of proteins, notably he obtained the first structure of a beta-lactamase, an enzyme that breaks down penicillin class antibiotics and is a primary mechanism of resistance to those drugs. His work in computational studies of protein structure and function began in earnest after taking up his position in Maryland, and has included proofs that protein folding is formally chaotic and an NP hard problem, and the development of Monte Carlo molecular dynamics, genetic algorithms and other methods for studying protein structure and folding. More recently, he has developed machine learning and related methods for the analysis of the relationship between human genetic variation and disease, including deep learning and graph-based approaches for representing disease mechanisms.
He is an author on more than 150 scientific publications.
John earned his BS in Physics at the University of London and his PhD in Molecular Biophysics at the University of Oxford.
Minutes
On September 24, 2021, by Zoom webinar broadcast on the PSW Science YouTube channel, President Larry Millstein called the 2,445th meeting of the Society to order at 8:02 p.m. EDT. He welcomed new members, and the Recording Secretary read the minutes of the previous meeting.
President Millstein then introduced the speaker for the evening, John Moult, Professor at the University of Maryland’s Institute for Bioscience and Biotechnology Research. His lecture was titled, “The First Serious Problem Solved by AI: How Proteins Fold to Become the Machines of Life.”
Moult began by relating his talk for the evening to the lecture delivered to the Society in 1999, which was also about doing community science. He then addressed the “provocative” title of his talk, stating that protein folding is an incredibly important problem that humans cannot solve without artificial intelligence.
Proteins are essential to most biological functions. The sequencing of a particular protein alone does not indicate the protein’s function. But, the sequencing of positively and negatively charged amino acids will cause the resulting protein to fold into a particular, highly ordered shape. These shapes can be reduced to a two-dimensional image.
There are approximately 1,000 common folds and a significant number of less common folds, produced by unique amino acid combinations. The novel shapes of differently folded proteins create surfaces which can be complementary to the surfaces of other molecules. Moult said these combinations are key to the whole of biology and illustrated his point by explaining the work of Osnat Herzberg.
Since the mid-20th century, scientists have known that if they could compute the various amino acid combinations, they could predict new proteins and their folded shapes. This problem has proven difficult because of the huge search space to find the right fold, a frustrated search landscape, and the finely balanced energy minimum of a given protein.
Scientists have taken essentially four different approaches to the folding problem based on structure principles, search methods, physics, and evolutionary relationships. Most approaches have failed. So far, only deep learning has successfully and efficiently produced models to predict protein structures.
Scientists began using computers tackle the folding problem in the 1980s and 1990s. Those efforts produced significant optimism, but few results. Moult said computer modeling lacked the rigor of the real world, could not be effectively peer reviewed, and thus could not solve the real world problem. To address that failing, Moult and his colleagues, including Christoph Fidelis, introduced the Critical Assessment of Structure Prediction (CASP).
CASP is roughly analogous to a clinical trial, by going to experimentalists every two years to inquire about their then-current non-public research. The structures those experimentalists are working on are then sent to other researchers in the community, who are invited to produce computed models which are later compared to the experimental results. Around 100 groups of researchers participate.
Moult then discussed a sampling of CASP results. At times, CASP has shown progress in solving the folding problem and, at others, progress has appeared to stall out. CASP 2 models in 1996 had only 8% contact precision compared to experimental results, quickly doubling in 1998 and reaching 20% in 2000. But that rate of improvement quickly tapered, reaching only 25% contact precision by the time of CASP 11.
Most recently, contact precision has leapt, reaching 47% in CASP 12 and 70% in CASP 13. These dramatic improvements are credited to advancements in deep learning, by which model neural networks “learn” from two-dimensional images of protein structures to predict new protein folds.
Early deep learning used neural networks to perform convolutional feature extraction to piece together a complete prediction network. These early efforts produced accuracy rates around 60%. By 2020, deep learning improved accuracy to 85%, principally led by research groups Alpha Fold and Deep Mind.
Deep Mind’s method starts by creating a contact map and then in a second stage of the network, inputs contact maps to output atomic coordinates. The method also uses attention learning, by which part of the network “interrogates” the process to identify where there is greatest information flow to construct a set of weights for where to relate things. Deep Mind also incorporates features of the physics approach to the problem to simplify the network structure.
With these improved models, experimentalists have been able to quickly move through many impasses. Moult predicts the rapid developments will produce breakthroughs in rare disease research such as sickle cell anemia and contribute significantly to cancer research. Pharmaceutical research will also benefit by understanding how drugs bind to proteins. He then described how the deep learning models have been applied in SARS2 research.
These new models will also allow scientists to produce artificial proteins to produce better commercial products, like soaps.
Moult concluded with his thoughts on how to judge how “intelligent” any particular machine really is. He asserted that “intelligence” can be measured by how well a machine can generalize and apply itself outside the data on which it was trained.
The speaker then answered questions from the online viewing audience. After the question and answer period, President Millstein thanked the speaker, made the usual housekeeping announcements, and invited guests to join the Society. President Millstein adjourned the meeting at 10:16 p.m.
Temperature in Washington, D.C.: 18° C
Weather: Clear
Concurrent Viewers of the Zoom and YouTube live stream, 44 and views on the PSW Science YouTube and Vimeo channels: 353.
Respectfully submitted,
James Heelan, Recording Secretary